PrivacyUmbrella: Anonymizing medical data
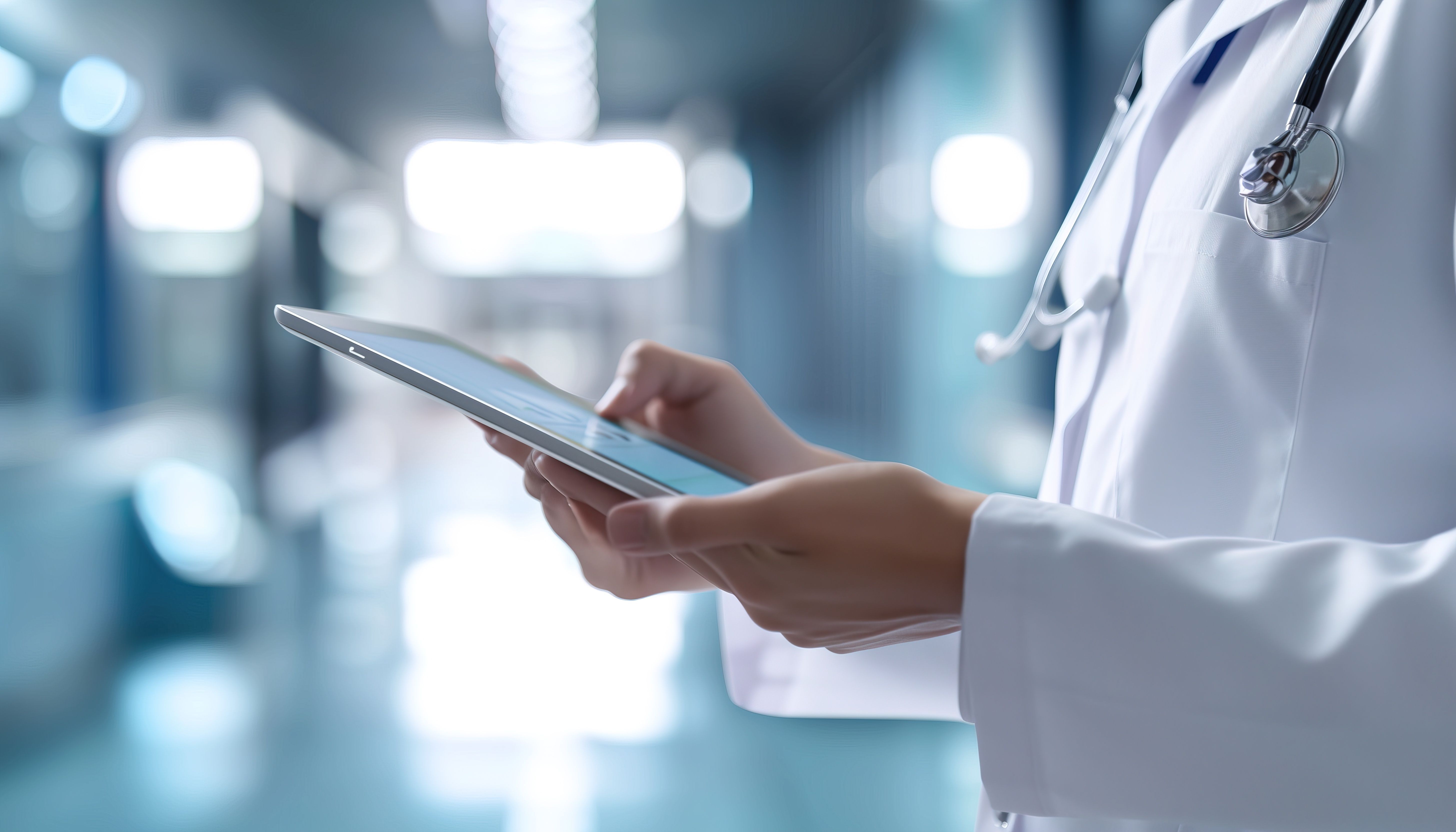
Health data is becoming more detailed all the time and is accessible to many parties. However, this means it is also vulnerable to attacks that breach the privacy of individual patients. Anonymization processes can protect patient privacy by ensuring it is no longer possible to identify individuals in data sets. However, protecting data is not the only challenge here; it is also important to ensure that the data being shared is sufficiently informative for data analysis processes in the context of personalized medicine — and to enable machine learning on a large scale.
In the PrivacyUmbrella project, Fraunhofer ITEM scientists are collaborating with the university hospitals in Frankfurt and Mainz and MCS Data Labs GmbH with the goal of achieving more reliable anonymization using a combination of different established techniques, while also maximizing usability for data analysis at the same time. The project is being financed by the German Federal Ministry of Education and Research (BMBF) and through NextGenerationEU funding.