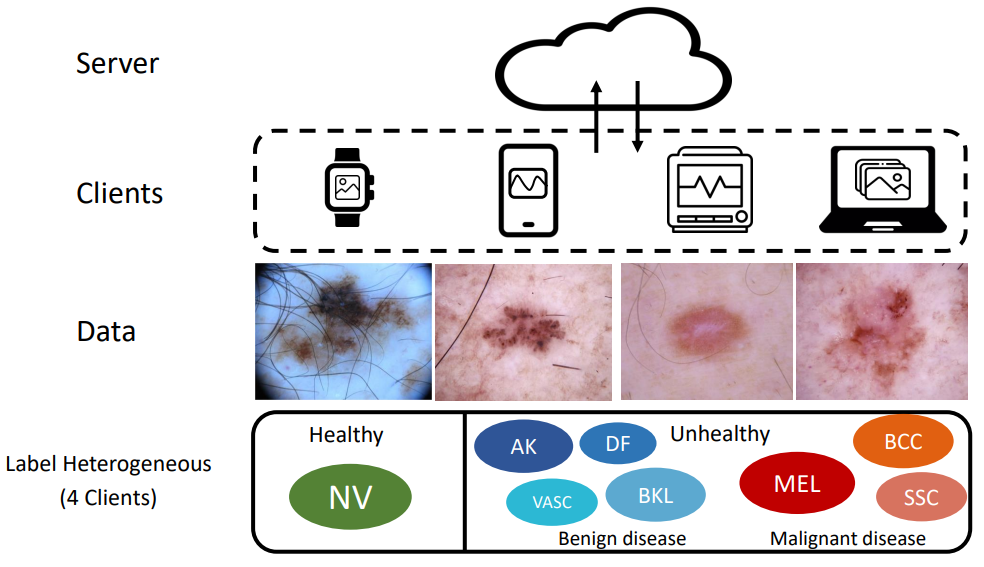
Over the past decade, the field of deep learning has experienced exponential growth. However, its use poses risks regarding data protection and privacy. Federated learning enables multiple independent devices to collaboratively train a global model without exchanging private data, thereby ensuring data privacy. However, users’ varying requirements for machine learning frequently lead to differences in data annotations, in other words, different ways of labeling or categorizing data within a dataset. This affects the overall performance of the system.
Researchers at Fraunhofer ITEM have developed a multi-task federated learning software as part of the PrivacyUmbrella project, implementing knowledge distillation. Knowledge distillation is used to compress large, highly accurate models into smaller, more efficient ones. This approach aims to integrate both heterogeneous devices and heterogeneous labels to train a global classification model. Experimental results using the publicly available dermoscopy dataset ISIC2019 demonstrate that the framework significantly reduces computational and communication costs for resource-constrained clients while allowing high-performance clients to flexibly select neural networks. Various clients handle different classification tasks for the eight skin lesion types in the ISIC2019 dataset: melanocytic nevus (NV), actinic keratosis (AK), vascular lesion (VASC), benign keratosis (BKL), dermatofibroma (DF), melanoma (MEL), squamous cell carcinoma (SCC), and basal cell carcinoma (BCC).
- Client 1 uses a simple binary classification system, categorizing images as "healthy" or "unhealthy" to quickly identify skin conditions that require further attention.
- Client 2 employs a three-level classification system: "healthy," "benign," and "malignant," which is relevant for distinguishing;” the distinction between benign and malignant conditions is relevant for detailed analysis in the clinical setting.
- Client 3 refines the classification further by categorizing images into four groups: MEL, BCC, SCC, and "Other." This is particularly useful for specialized analyses that differentiate between various forms of skin cancer.
- Client 4 utilizes the most detailed classification system, categorizing images into eight different skin lesion types according to the ISIC2019 dataset. This system supports comprehensive diagnostic processes and precise treatment planning.
Experimental results show that clients utilizing knowledge distillation achieve better performance. Future research will focus on validating the robustness and versatility of the approach by applying the framework to additional datasets and scenarios.